How Generative AI Is Transforming Medical Education
Harvard Medical School is building artificial intelligence into the curriculum to train the next generation of doctors
- 11 min read
- Feature
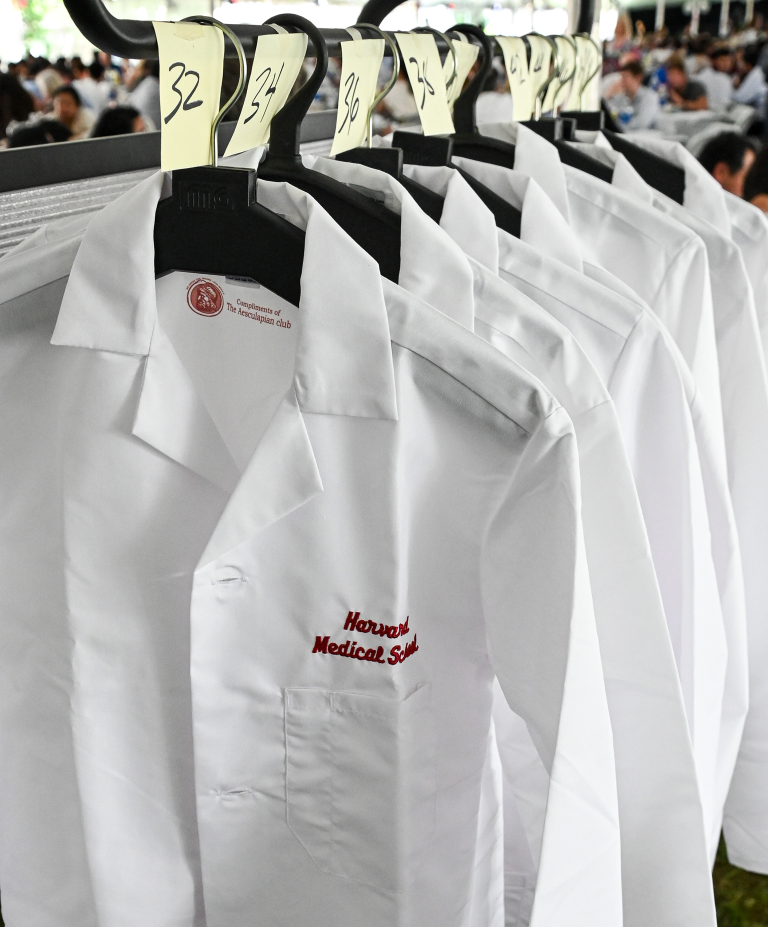
Within a few weeks of its public launch in November 2022, ChatGPT was already beginning to feel ubiquitous, and Bernard Chang, MMSc ’05, was thinking about what that meant for the future of medical education. “Maybe once every few decades a true revolution occurs in the way we teach medical students and what we expect them to be able to do when they become doctors,” says Chang, HMS dean for medical education. “This is one of those times.”
By 2023, studies found that the initial public version of ChatGPT could perform at a passing level on the U.S. Medical Licensing Exam. A more powerful version of ChatGPT, released in March 2023, exceeded the performance of medical students, residents, and even practicing physicians on some tests of medical knowledge and clinical reasoning, and today there are a number of large language models that match ChatGPT’s abilities. So how will this affect today’s medical students — and the institutions educating them?
Chang says that the last such revolution in medical education occurred in the mid-1990s, when the internet became widely accessible. “Initially we just played games on it,” he says. “But it soon became indispensable, and that’s what’s happening with generative AI now. Within a few years it’s going to be built into everything.”
HMS is getting a jump on this shift by building generative AI (also called genAI) into the curriculum today. “The time is right to respond to this call,” Chang says. “We didn’t hold back and wait to see what other schools are doing, both because as an institution we wanted to be at the forefront of this and because it’s the right thing to do for our students.”
Incorporating AI
Among the changes incorporated this fall is a one-month introductory course on AI in health care for all incoming students on the Health Sciences and Technology (HST) track. “I don’t know of any other med school doing that,” says Chang. “Certainly not in the first month.” The course examines the latest uses for AI in medicine, critically evaluates its limitations in clinical decision-making, and crucially, he adds, “grounds students in the idea that medicine is going to be different going forward. In this day and age, if they want to be a physician-scientist or a physician-engineer, which is the goal of the HST curriculum, they won’t just need to be a good listener and a good medical interviewer and a good bedside doctor. They’ll also need good data skills, AI skills, and machine-learning skills.” About thirty students each year enroll in the HST track, and many of them will get a master’s degree or PhD in addition to their MD.
A PhD track that starts this semester, AI in Medicine (AIM), is taking AI-integrated education even further. “Bioinformatics students were increasingly saying they were excited about AI and asking if we could offer a PhD in it,” says Isaac Kohane, the Marion V. Nelson Professor of Biomedical Informatics and chair of the Department of Biomedical Informatics in the Blavatnik Institute at HMS. “We didn’t know how much demand there would be, but we ended up with more than 400 applications for the seven spots we’re offering.”
“As with any big technological eruption,” Kohane says, “for a few years there will be a huge gap in the workforce. So we want to train researchers who know a lot about medicine and understand real problems in health care that can be addressed by AI.”
Also to that end, HMS has opened a third avenue for medical students and faculty who are interested in the technology: the Dean’s Innovation Awards for the Use of Artificial Intelligence in Education, Research, and Administration, which were announced last year and offer grants of up to $100,000 for each project selected (see “Advancing Innovation in Medical Education,” below). “These grants really show HMS is leading the way in trying to integrate these amazing new tools into the way we work and learn,” says Arya Rao, an MD-PhD student and a co-recipient of an award to study AI for clinical training. “I’m grateful to have this experience to take forward into my medical career.”
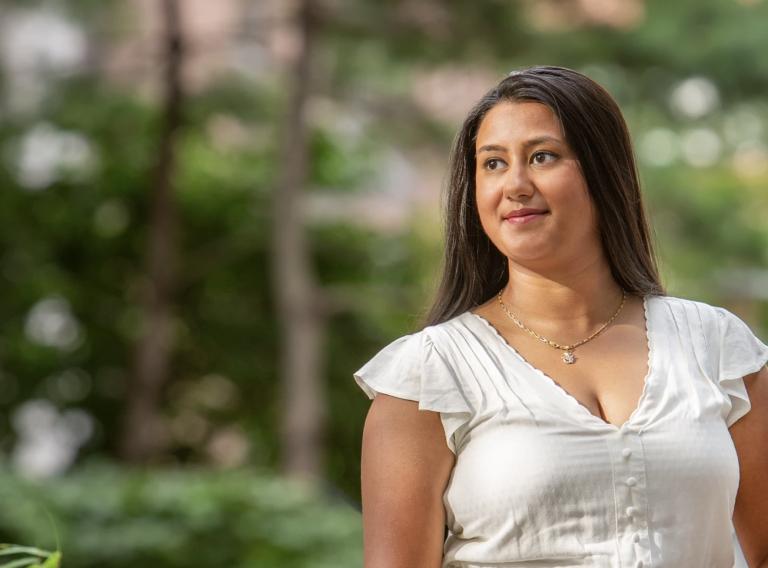
Hospitals affiliated with HMS are also incorporating AI into their clinical workflows. Brigham and Women’s Hospital, for example, is testing the use of an ambient documentation tool that takes clinical notes so that doctors can spend more of their time interacting with patients. As these kinds of tools are implemented, Chang says, they will allow students to focus on talking to patients “instead of constantly turning away to look at a screen. It will also help them shift sooner to higher levels of learning and more advanced topics and things we want our doctors to do, like listen.”
“GenAI is often viewed as taking the humanity out of communication,” says Taralyn Tan, the assistant dean for educational scholarship and innovation within the Office for Graduate Education. “But I actually see it as being a mechanism to reincorporate a human dimension to clinical practice by taking the burden of many administrative tasks off of doctors.”
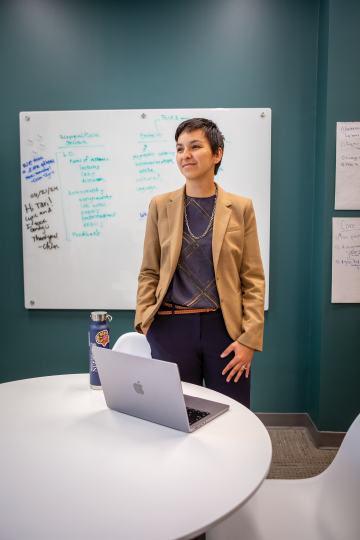
Rao agrees. “The real beauty of medicine, the reason to be in it, is the bonds you’re able to make with patients,” she says. “If you look at the amount of time doctors spend digging through medical records and writing notes, it’s hours and hours a day. AI can free up some of that time so we can devote it to what we’re really here for, which is helping people.”
Richard Schwartzstein, chair of the Learning Environment Steering Committee and the Ellen and Melvin Gordon Distinguished Professor of Medical Education, sees the value in corralling record-keeping and other such duties, but he warns that taken too far, AI use may lead to deficits in a student’s preparedness. “We need to put it in the context of real-world bedside medicine and how you work as a physician by emphasizing reasoning and critical thinking,” Schwartzstein says. “What does the bedside clinician use it for well? What does the clinician have to be wary of? What does the clinician still need to be good at to use AI appropriately?”
Schwartzstein points out, for example, that AI can help doctors track down pathogens from places around the world that a patient may have been exposed to but that the physician is unfamiliar with. “I can do that now just with the internet,” he says, “but AI can do a broader and faster search. One of the drawbacks, though, is that it doesn’t tell you what sources it’s looking at, so you can’t be sure if the information comes from a journal you trust.”
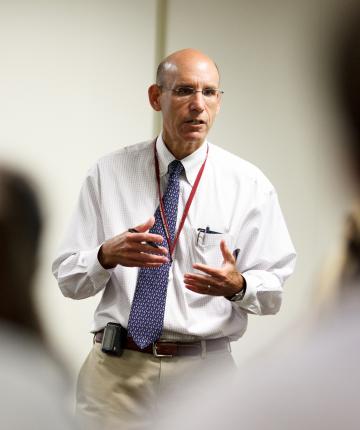
Double-checking AI’s results is key, he says, as is being able to match the options it provides with a patient’s actual symptoms and history. “AI isn’t good at problem-solving, which is one of the toughest parts of medicine,” Schwartzstein notes. A study from researchers at HMS and Beth Israel Deaconess Medical Center found that although ChatGPT was accurate when making diagnoses, it made more errors than physicians in reasoning — tasks like considering why certain questions should be asked rather than just what to ask — than its more experienced human counterparts, doing better than residents but not attending physicians.
Schwartzstein says another area where students may be susceptible to overusing AI is in analyzing lab data. “Interpreting tests and working in inductive mode helps them learn critical thinking,” he says. “The majority of malpractice cases arising from possible diagnostic error are not weird cases. They’re basic cases that people make mistakes on — thinking errors. So while using AI for a case like that would be great for a nurse practitioner in an under-resourced area without the backstop of a physician nearby, it would be problematic for a physician to not have that training and competence in thinking skills.”
Once doctors have some years in practice behind them, though, “having a consistent AI agent overseeing our actions and catching errors would be a huge win,” Kohane contends. “Sometimes rookie errors are made by experienced physicians because they’re tired or not feeling well, so having our work checked by AI might significantly improve mortality and morbidity in hospitals.”
Practical applications
But isn’t AI, too, famously prone to error? ChatGPT’s “hallucinations” — such as providing a detailed but very wrong answer by glossing over the obvious error in a prompt like “What is the world record for crossing the English Channel entirely on foot?” — are the stuff of memes. This problem is expected to improve over time, says Kohane, but even today, he notes, “AI makes different kinds of errors than the ones humans make, so it can be a good partnership.” Not only is the underlying technology improving, he notes, but it also massively expands the data pools physicians can draw on to arrive at diagnoses. For instance, a machine-learning model trained on close to one million electrocardiograms was able to perform as well as or better than cardiologists in diagnosing thirty-eight types of conditions. “Imagine what that could be in the hands of primary care doctors,” Kohane says.
Such gargantuan datasets can be made even more comprehensive when they’re supplemented by electronic health records (EHRs) and input from patient wearables, Kohane points out. “GenAI doesn’t have to draw only from trials and medical journals,” he says. “If real-life data is gathered with consent and transparency, that extra information can help physicians see things they might not see otherwise.”
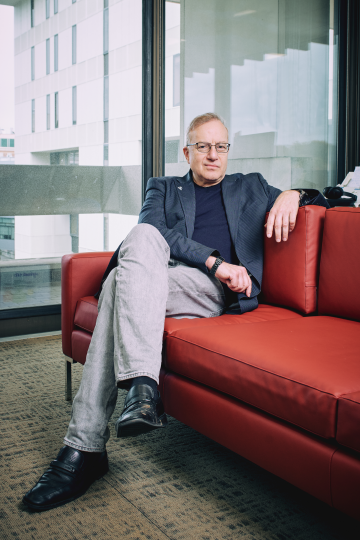
EHR data is also being used in a pilot project funded by the Dean's Innovation Awards with the goal of identifying gaps in a student's learning. “When they’re on the wards,” says Chang, “students can only learn from patients who happen to be in the hospital at that time." But the tool being developed will have access to both curriculum objectives and EHR data, allowing comparisons between learning objectives and the patients a student has actually encountered. Within a few years, Chang believes, such use cases will be commonplace. “Before going into rotations, students will access an app on their phones that will say, ‘Good morning, I suggest you see these three patients,’ because those patients represent gaps in the students’ knowledge.”
The problem of bias in AI training data is also well documented. And as Schwartzstein and colleagues point out in a paper published in the journal CHEST, not only is AI itself prone to reproducing the biases inherent in the human-generated materials it learns from, but also at least one study has shown that that loop can circle back and pass AI biases on to humans.
At the same time, there is evidence that feedback can work in the other direction as well. A recent study from Brigham and Women’s shows that including more detail in AI-training datasets can reduce observed disparities, and ongoing research by a Mass General pediatrician is training AI to recognize bias in faculty evaluations of students.
“There are a lot of biases no matter where the information is coming from,” says Tan, “so we have to keep an attentive eye on that. But AI can be a useful tool in our tool kit for promoting equity in education if we can leverage it in synergistic ways — putting in specific articles, citations, tools we know are effective, for example, and asking it to draw from the resources that reflect the latest in the field while remaining aware of these issues.”
Part of the solution then, is being aware of the data used to create AI tools. Chang mentions HMS “tutorbots,” which are trained on homegrown curricula. “We’re using ChatGPT as the engine,” he says, “but constraining it using the language and the course information we’ve given it. If we didn’t, what would be special about coming to HMS?”
Given all the changes happening, what will be special about an HMS degree when it comes time for this year’s cohort to move on?
If the students in the AIM PhD program graduated today, “they would be immediately approached with top job offers in all the competitive hospitals and universities,” Kohane says. “I would estimate that 60 percent of the graduates will go into industry. But when they get out in five years or so they’ll find plenty of green fields in academia and research, too.”
The reason for that lies, in part, in the adaptability of students trained in these technologies, says Tan. “It’s hard to predict how far this will go,” she says. “But tomorrow’s most successful physicians and researchers will be the ones who can harness genAI for innovation and strategic planning. The people who come up with solutions will be the ones who are using these tools.”
Advancing Innovation in Medical Education
In March 2024, HMS announced thirty-three recipients of the Dean’s Innovation Awards for the Use of Artificial Intelligence in Education, Research, and Administration. Below is a sample of the projects related to medical education.
The future patient persona: An interactive, large language model–augmented Harvard clinical training companion
Arya Rao, Marc Succi, and Susan Farrell
Providing opportunities for students to practice their clinical skills on standardized patients is an important part of medical school, says Rao. When the “visit” is over, students are graded by both the actor portraying a patient and their professor on their clinical reasoning, communication skills, and more. But the expense and time this takes can limit these opportunities. So Rao, Marc Succi, an HMS assistant professor of radiology at Mass General, and Susan Farrell, associate dean for assessment and evaluation and director of the comprehensive clinical skills OSCE exam, are developing customized large language models that can serve as standardized patients. They are reinforcing these models, which they call SP-LLMs, with material specific to the HMS curriculum. Students will be able to interact with the models using both text and voice, gathering patient histories, obtaining diagnostic information, and initiating clinical management, all while practicing their communication skills.
“One nice feature is that when the visit is over,” says Rao, “the SP-LLM also provides the student with feedback on the encounter, acting as both patient and preceptor. Since the tool is available anytime, anywhere, students can get a lot more practical experience before they start seeing real patients.”
Development of a generative artificial intelligence grading and learning tool
Greg Kuling, Jay Vasilev, Samantha Pullman, Randy King, Barbara Cockrill, Richard Schwartzstein, and Henrike Besche
HMS’s Pathways curriculum track emphasizes independent study and case-based collaborative classwork. Schwartzstein and colleagues have developed a system that enables bulk auto-grading of short-answer questions to summarize students’ strengths and weaknesses, identify conceptual challenges, and suggest tailored teaching strategies. It takes Schwartzstein, who chaired the steering committee that developed the Pathways curriculum in 2015, about eight hours to grade responses to a single open-ended question for all 170 students in a class, not including providing feedback. “I can’t possibly do that with homework,” he says, “but it would be really helpful to them if AI could.” Streamlining the process, he adds, will allow students to do more exercises and hence “get more practice at figuring out whether they’re correctly applying the principles they’ve learned to case studies.”
Harnessing generative AI to create learner-centered and evidence-based course syllabi
Taralyn Tan and Krisztina Fischer
Tan and Krisztina Fischer, an HMS assistant professor of radiology, part-time, at Brigham and Women’s, are studying the use of AI in Tan’s Teaching 100 course to develop and pilot a tool that uses generative AI to create syllabi, with the goal of having it adopted by other HMS faculty. In the course, Tan’s students first try to create learner-centered, evidence-based syllabi components on their own, and then they work with AI to do the same thing. “The class has a very meta dual purpose,” Tan says, “because the students are experiencing it both in their own teaching and from a learner’s perspective.” Tan also allows her students to use AI in the classroom outside of this capstone assignment. “The most common response I get when I ask about this is that they didn’t know how to use it,” she says. “So that speaks to the need for basic competencies for engaging our learners with it.”
Elizabeth Gehrman is a writer based in Boston.
Images: Steve Lipofski (white coats); Gretchen Ertl (Chang and Schwartzstein); John Soares (Tan and Rao); Peter Gumaskas (Kohane)
Correction: This article was updated to clarify the status of the project using EHR data to identify gaps in student learning. It is a pilot project still under development.
Research at Harvard Medical School hangs in the balance due to the government’s decision to terminate large numbers of federally funded grants and contracts across Harvard University.