What Computer Vision Models Reveal About Human Brains
AI models designed to identify objects offer surprising clues about how we see and how we learn
- 6 min read
- Interview
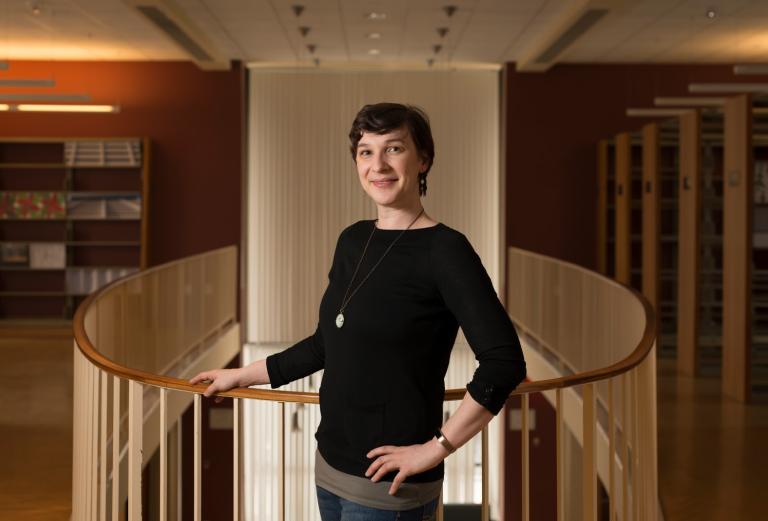
Talia Konkle
About 10 years ago, as computing power and the increasing availability of digital images led to major advances in computer vision models that use artificial intelligence, scientists like Talia Konkle noticed something strange: the models were working like human brains in surprising ways.
“These models weren’t designed to predict brains,” says Konkle, a Harvard University professor of psychology. “They were designed to take in images and tell us what’s there.” But when they zoomed in to examine the models’ stages of processing, scientists realized that the computers were learning to recognize features in much the same way humans do, using layers of neurons. Vision scientists started comparing brain and computer responses to the same images, discovering that the models could actually be used to predict how brains function.
“All of a sudden we have completely new tools to ask fundamental questions,” says Konkle, who is part of a growing community of researchers working at the intersection of neuroscience and AI. These questions include how the human visual system transforms patterns of light into meaningful objects and scenes and decades-old debates about how we learn from the world.
Harvard Medicine’s associate editor, Molly McDonough, talked with Konkle about what computer vision models are revealing about the human visual system.
Let’s start with human vision. Why are there so many lingering mysteries about how it works?
The problems of vision are primitive and deep, but the machinery of the brain does such a good job of making it feel effortless that we take for granted that we can see the world. We think that we see in full color and high resolution, but that’s all in our head. We get imprecise, sparse measurements, and a lot of it is filled in through internal mechanisms. It’s amazing!
Vision is a computational problem. Patterns of light are the input. They hit receptors at the back of the eye, leading to brain signals that you eventually use to create meaning and recognition. The output is what you identify from a list of possible things. It’s really hard to understand how brain tissue is able to recognize a specific thing from all the possible things.
So in that sense, the problem of human vision is kind of like the problem of computer vision.
Yes, and the architectural similarities between human and computer vision are not arbitrary. The smallest building blocks of computer vision models are inspired by neurons. There are many ways you can connect these building blocks, but the technologies commonly used in computer vision have a macroscale architecture that is hierarchical and directly inspired by the human visual system.
The biological visual system is still far, far, far more complex than these models by so many orders of magnitude. You can make a solid case that the models are nothing like brains. But if you fly higher and you look at how computer models and human brains process information, there are some similarities.
Once we started to realize how much computer vision models were acting like brains, a host of new questions opened up: Why are the two matching so well? Can we improve models by fitting them to neural data? Can we design new models that are even more predictive of the brain? And, finally, now that computers can recognize objects, what should their next visual tasks be?
Thus the field of NeuroAI was born, with the goal of exchanging insights between computer and vision science. This led to some deep insights into how the biological visual system must be working, which is really cool.
Can you give an example?
One comes from advances in what’s called self-supervised learning. The first computer vision models were functions; they’d take an input and give an output. You take an image and you tell the computer which of a thousand categories it’s in. It requires labeling, which is a big hurdle. But a few years ago, people figured out that computers could learn from images without needing to know those labels at all.
It's actually really simple. The basic idea is that rather than trying to learn different categories, like dogs and cats and shoes, the computer just learns to distinguish everything it sees. It doesn’t need to know the categories. It just needs to know that this is a picture of something and it’s different from other things.
What’s magic about this, it turns out, is that pictures of cheetahs, for example, all end up kind of near each other in the computer’s mind, and pictures of dogs end up near each other, and shoes end up near each other, without the models ever needing to know about cheetahs or dogs or shoes, or even that they’re categories at all.
The similarity structure of the world makes its way through the network, so that you get this meaningful clustering of things without even needing to know that there are things at all.
OK, but say you wanted the model to tell you if something is a cheetah. Would you then show it a cheetah and say, here’s a cheetah, and it could connect it to all the cheetahs it had already seen?
Yes, exactly. Now it’s an indexing problem, it’s a little lightweight operation to teach the computer the word “cheetah.” If you want it to learn a new category, you just plug it in. That was a big discovery. It meant that we now have systems that can pick up on the structure of the world and map it in a useful way without needing to label all the data.
The parallels we see with human brains in these self-supervised models are just as strong as in the previous category-
There’s been a lot of debate about the innate structure of the mind. Some argue we start out pre-wired to recognize different categories, but others think that no, you can pick up the categories from the input. And these models are giving us new ways of articulating how you actually get off the ground learning: how much is built in, and how much can just emerge. We keep being delightedly surprised by how you can get so much rich structure from really simple learning rules. It just kind of naturally emerges.
I’m reminded of my one-year-old daughter, who seems to soak up knowledge like a sponge.
In the past couple of years, people have wanted to know what you can learn from the eyes of a baby. So researchers have been making these datasets by putting a little camera on a baby’s head and collecting video of what the babies see and audio of the words they hear. As an example, Brenden Lake at NYU basically created a computer model that learned through the eyes and ears of his child. And the model learned good representations. It learned to categorize different objects from just really simple learning rules and visual and auditory input. Understanding what we need in order to learn and what the constraints are on learning is really fun as a scientific frontier.
How have these technologies opened up new possibilities for your research?
My work focuses on the late stages of visual representation, when we start to recognize things — when there are bits of brain with neurons that will fire strongly for pictures of faces, bodies, and words, but not for other categories of things. But there can’t be a neuron for every possible thing we could see. So how are other kinds of things represented across this swath of cortex that codes for objects? Some of my early work showed that object information is distinguished based on the size of objects. Through many empirical studies using fMRI, I mapped how different objects activate this cortex, and that led to some clear theoretical ideas, but we lacked rich computational models to see if those ideas were right.
Then came deep neural networks, a type of AI computer vision technology that learns from a rich variety of natural images to distinguish things from all other things. We immediately started doing the same scientific experiments we did on the human visual system, but this time on artificial visual systems.
Unlike with biological brains, where getting measurements and figuring out connections is time-consuming and often methodologically impossible, with deep neural networks we have access to every unit. We can measure responses to any image, we can create “lesions” to turn off specific units and see how the model breaks. It’s a neuroscientist’s playground.
Around the early 2000s, we figured out how to get human brain measurements from fMRI, which is my main methodology, and that led to a bunch of advances. But such new methods that really break open a field don’t come around often. We’re just at the beginning of this new method that’s making a whole host of new research questions possible. It’s a really good time to be a vision scientist.
Image: Anna Olivella