Did AI Solve the Protein-Folding Problem?
Now that AlphaFold has tackled biology’s greatest challenge, scientists can turn to even bigger questions
- 12 min read
- Feature
Oxyhemoglobin, the hemoglobin molecule in its oxygenated state, as illustrated by Irving Geis.
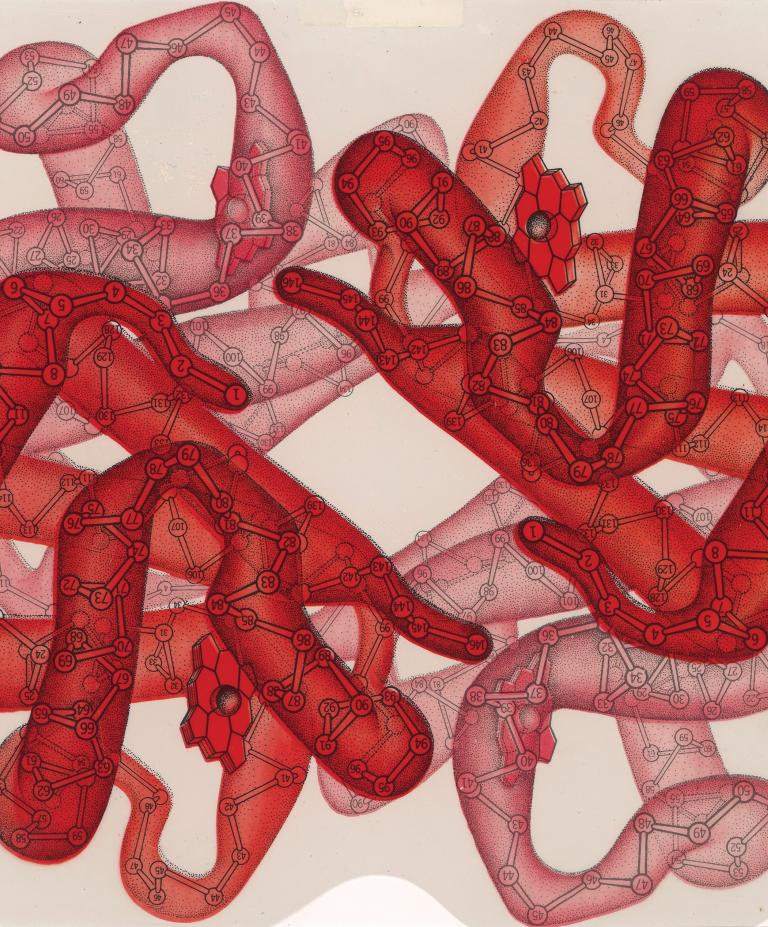
Oxyhemoglobin, the hemoglobin molecule in its oxygenated state, as illustrated by Irving Geis.
It started with a slab of sperm whale meat.
It was the early 1950s, and two British molecular biologists were trying to achieve a feat that had thus far eluded scientists: creating a three-dimensional model of a protein molecule.
Like other biologists at the time, John Kendrew and Max Perutz knew that proteins are essential building blocks of life, responsible for catalyzing every kind of reaction in the body. They suspected that the structure of a protein would offer clues about its particular function. But determining a protein’s shape was no small task. It involved a time-consuming technique called X-ray crystallography: growing crystals out of protein from tissue samples and then bombarding those crystals with X-rays to measure the angles at which rays bounce off individual atoms to piece together the molecular structure.
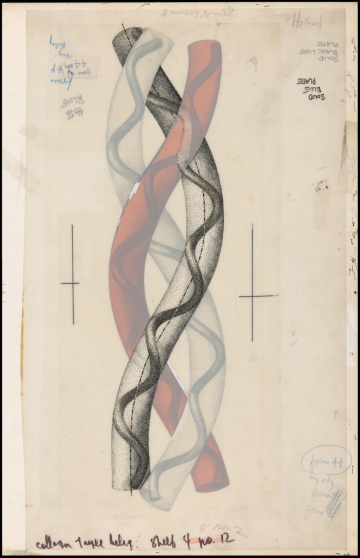
Kendrew had set out to model myoglobin, a protein that stores oxygen in muscles. After failing to spur sufficient crystal growth from penguin, tortoise, and porpoise meats, he finally found success using a nearby lab’s stash of whale flesh. (Sperm whales, promoted as an alternative source of meat in the U.K. during World War II, need abundant myoglobin to store oxygen during deep sea dives.) Aided by a squad of workers performing calculations and a computer weighing no less than six tons, Kendrew and colleagues finally unveiled the very first three-dimensional model of a protein molecule in 1958. The whole effort had taken more than two decades.
Fast-forward to today, and Nazim Bouatta, an HMS senior research fellow in systems biology and systems pharmacology, pulls up a webpage, enters a sequence of letters into an input form, hits a play button, and scrolls down. Almost instantaneously, a squiggly blue model of a molecule appears. This protein molecule has never been modeled in a lab using techniques like the ones Kendrew employed. Instead, it’s being pieced together using an artificial intelligence model called AlphaFold2. What took Kendrew decades now takes seconds.
The system Bouatta is displaying — an AI model that predicts the structure of protein molecules — may just be the most-hyped AI technology in science. Since AlphaFold2 debuted in 2020, journalists have touted it as “the most important achievement in AI, ever,” and “biology’s holy grail.” “One of the biggest problems in biology has finally been solved,” proclaimed a Scientific American headline. Two of its creators, Demis Hassabis and John Jumper of Google DeepMind, even won the 2024 Nobel Prize in Chemistry for their work.
But how much of that very big biology problem did AI solve, and what does that mean for the scientists who have spent their careers trying to crack it? As they turn to the questions that the AI models haven’t answered, researchers like Bouatta are provoking bigger conversations about the roles of computers and humans in the discovery process — including how AI could transform the definition of science itself.
The protein-folding problem
To understand the mystery that AI is purported to have solved, it helps to dwell on that myoglobin model for a minute. Although Kendrew and Perutz went on to win a Nobel Prize for the work, Kendrew himself seemed underwhelmed with the model (shown below), admitting that “it could not be recommended on aesthetic grounds.” Unlike the DNA double helix, first modeled in the same U.K. lab just a few years earlier, the myoglobin molecule was not elegant and symmetrical. Colleagues compared it to “abdominal viscera” and “worms”; writing of its appearance in a museum years later, a Guardian reporter dubbed it the “turd of the century.”
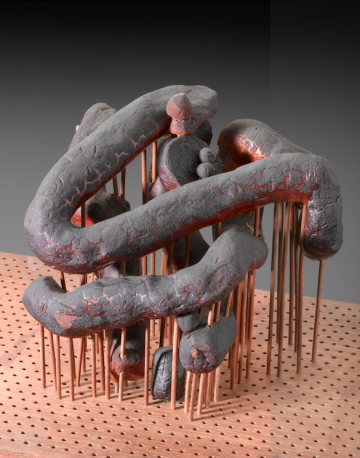
Jokes aside, scientists soon realized that the first few protein models would not offer a blueprint scientists could use to model the structure of all proteins. “One of the sad aspects of this discovery is we cannot see anything from the pattern,” said physicist Richard Feynman during a 1964 lecture. “We do not understand why it works the way it does. Of course, that is the next problem to be attacked.”
What Kendrew and Perutz’s work did do was lay out the experimental techniques researchers could use to piece together other proteins — which is exactly what they did, protein by painstaking protein, over the ensuing decades. Advances in imaging technologies helped speed the process along, but even today, it typically takes weeks to months to map out a single protein in a lab. Around 200,000 proteins have been modeled this way. “It’s a lot,” Bouatta says, “but if you put it in perspective with respect to the number of proteins that exist in nature, it’s a drop in the bucket.”
Helpful insights emerged over time. Scientists learned that proteins start out in cells as long chains of amino acids. In the 1960s, Christian Anfinsen, PhD ’43, proved that the sequence of these amino acids is like a recipe for how the protein will shift into its ultimate shape. “It starts as a floppy kind of spaghetti floating in water,” Bouatta says. “Then it starts folding.”
Thanks to advances in DNA sequencing, figuring out the makeup of those initial amino acid sequences has become a cinch. “You can just swab bacteria off the sidewalk and throw it into a DNA sequencer to get all the protein-coding regions of its genome,” says Nicholas Polizzi, an assistant professor of biological chemistry and molecular pharmacology at HMS. “So we have billions of protein amino acid sequences from natural organisms in DNA databases.” In other words, it’s easy to find out how a protein begins. But figuring out exactly how it will fold into its final shape is much tougher.
That’s because the transition from floating spaghetti to folded protein is mind-bogglingly complex. The way a protein’s atoms interact with one another is shaped by complex physical forces, the surrounding environment, and the number of amino acids in the protein. Even a small protein made up of, say, one hundred amino acids could theoretically fold into a dizzying range of shapes. If the process happened through random sampling, it could take 1052 years for a protein to find its most stable, lowest-energy state — longer than the age of the universe. Yet somehow, in real cells, many proteins fold within a thousandth of a second.
It starts as a floppy kind of spaghetti floating in water. Then it starts folding.
The so-called protein-folding problem thus ties together three related questions. What are the physical forces that transform a string of amino acids into a folded protein? How does it happen so quickly? And is there a way to predict how proteins will fold using their amino acid sequence alone?
Cracking the code
For a few decades, a group of researchers has been working to tackle this problem using computers. “At first, the paradigm was very physics-based,” says Mohammed AlQuraishi, an HMS fellow in therapeutic science and an assistant professor of systems biology at Columbia University. “You start with a protein sequence, you understand the types of energies involved in protein folding, and you try to emulate that on a computer.”
While working as a fellow in the laboratory of Peter Sorger, the Otto Krayer Professor of Systems Pharmacology at HMS, AlQuraishi was among the scientists first testing if another approach could work: Instead of baking physics into the computations, could an AI model just comb through data on amino acid sequences and the corresponding molecules modeled in labs and learn how to fold proteins on its own? That’s the general premise of deep learning. A model like ChatGPT, for example, isn’t explicitly taught vocabulary or grammar. Instead, it learns to recognize patterns by being fed huge quantities of text.
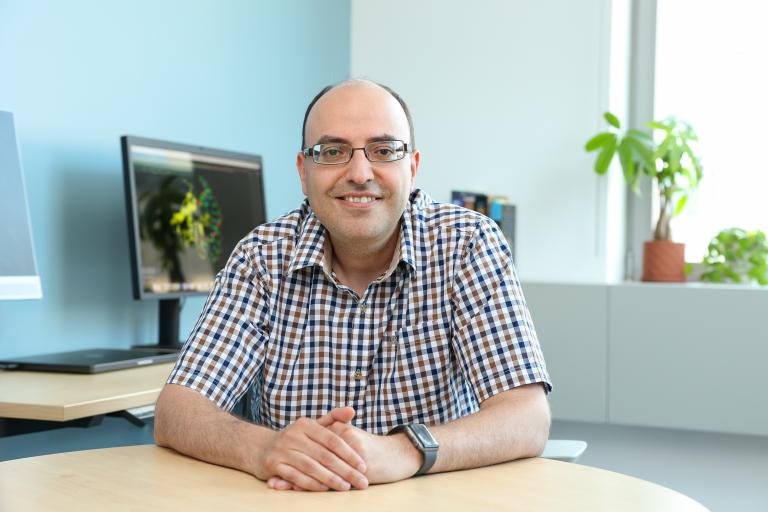
In 2018, AlQuraishi released an AI model using this approach that could make pretty good estimates of protein structures about six times faster than other existing methods. Another deep learning model developed in the lab of Debora Marks, a professor of systems biology in the Blavatnik Institute at HMS, came out several months later. At the time, AlQuraishi recalls, colleagues in the field were skeptical that this approach could ever be accurate enough. “The consensus was, ‘this is kind of cute, but ultimately you need physics to make this really work, right?’ ” he says.
The skeptics didn’t know it then, but a turning point was near. It arrived in 2020. Computational biologists had convened for a biennial competition, called the Critical Assessment of Structure Prediction, or CASP — akin to the Olympics of protein structure prediction. The contest works like this: Organizers release amino acid sequences for a number of proteins whose folded structures have already been modeled in labs using experimental methods but haven’t yet been made publicly available. Contestants then use computer models they’ve developed to predict how those proteins will fold based only on the sequences. Whoever’s model comes closest to the actual folded proteins wins.
The day before the awards, AlQuraishi sat at his computer — the event was virtual due to COVID-19 — and combed through results as soon as they were posted online. “It was pretty clear something profound had happened,” he recalls. An AI model called AlphaFold2, developed by researchers at Google DeepMind, had predicted protein structures with a level of accuracy no model had even come close to before. It would sweep the competition.
Like the previous model AlQuraishi developed, AlphaFold2 eschews a physics-based model in favor of deep learning. But another important quirk is built into its architecture. When fed an amino acid sequence, the model scans many other similar sequences found in nature, detecting changes in some sections of the sequences that coincide with changes in others. These patterns suggest that the changes evolved together and are thus likely to be close to one another in space when the protein folds.
Adding this step worked remarkably well. Within months, the number of predicted protein structures available to scientists shot up from 200,000 to 200 million. Importantly, AlphaFold2 could assign a confidence score to its predictions so that scientists knew how much to trust each one. Although not all of the predictions had a high confidence score, for the first time, a significant proportion of protein structures predicted by machine learning were as accurate as those developed in labs — accurate enough to have actual applications in biology.
Democratizing AI
The computers had become as good as the humans, and they were a heck of a lot faster. Cue the existential crises. “From the perspective of a scientist who wants to see progress, this was enormous,” AlQuraishi says. “But it was bittersweet to think, suddenly, it’s done.” He pondered the fate of his own research program, which he had mapped out years into the future to solve a problem that was now “eviscerated overnight.” Colleagues wondered if AI would spell the end of methods like crystallography and of structural biology itself.
But the initial shock gave way to curiosity. “What’s happened is a realization that now we go to bigger problems,” AlQuraishi says. “If we can get individual proteins solved, what about complexes? What about proteins interacting with other molecules?”
AlphaFold2 also had important limits. DeepMind released the source code needed for researchers to model other proteins, which was very useful for predicting a protein’s typical structure, but the AI model wasn’t built to predict mutations or to explain how proteins might interact with one another or with drug molecules. And DeepMind didn’t release enough of the code for researchers to retrain the model with different data or to innovate by adding new functions.
AlQuraishi and Bouatta knew they were at a crossroads. For researchers outside of Google to have access to the AI needed to tackle ever-bigger problems, they’d need to create something new: a fully open-source model inspired by AlphaFold2. They decided to make it happen. Collaborating with students — led by Columbia master’s student Gustaf Ahdritz, now a PhD candidate at Harvard — they released that model, called OpenFold, six months later.
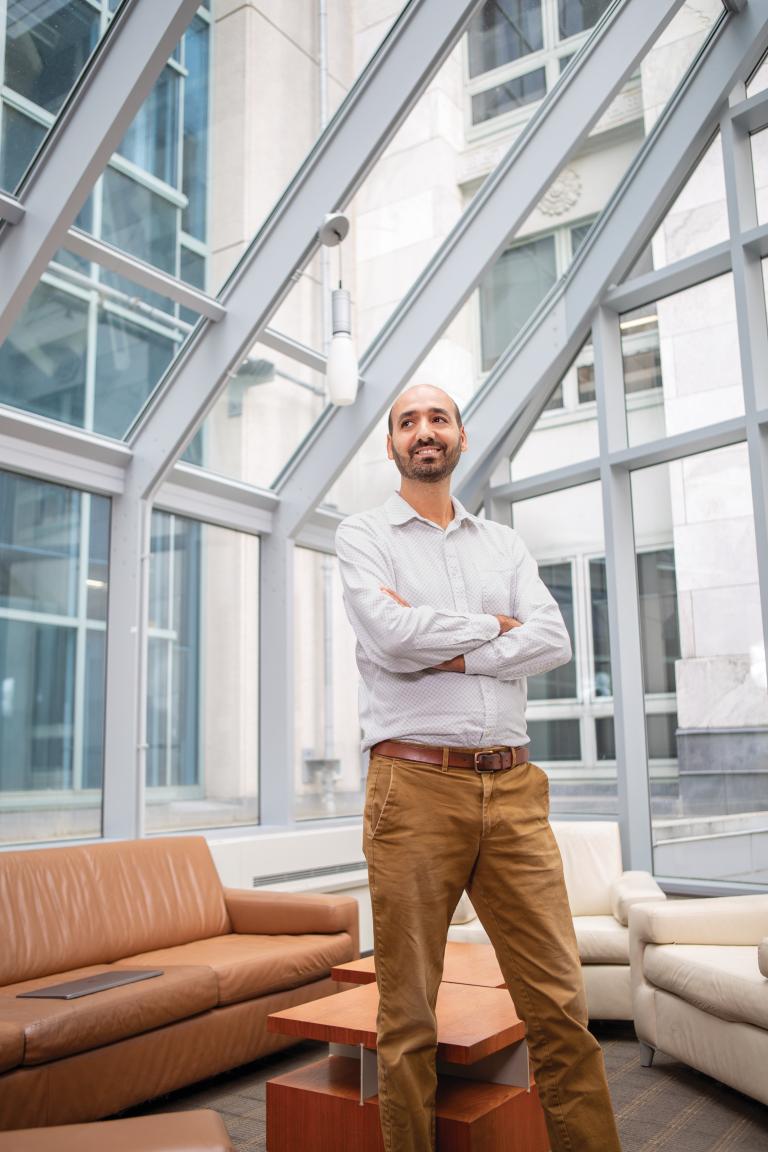
OpenFold was even faster than AlphaFold2 and just as accurate. And it could be retrained, which soon led to further advances. By combining OpenFold with a language model similar to ChatGPT, for example, Meta AI was able to release the structures of more than 600 million little-known proteins, like viruses that live deep in the ocean. And Bouatta is now working with research fellow Elena Rivas in Harvard’s Department of Molecular and Cellular Biology to use OpenFold to predict three-dimensional structures of RNA, another central challenge in biology.
Of course, researchers at DeepMind have continued plowing ahead. Earlier this year they released an improved model called AlphaFold3. Published in Nature, the new model made big strides in predicting how proteins could interact with one another and with potential drug molecules. But this time, DeepMind released far less of the code than it shared for AlphaFold2.
Polizzi, who uses both AI models like AlphaFold and experimental methods to explore how small molecules bind to proteins, was among a group of researchers who wrote a letter to Nature criticizing the journal’s decision to publish AlphaFold3 without the accompanying code that would let scientists evaluate and build upon the work.
“We were pretty disappointed with the way the paper was published, which skimped on some important aspects of peer review,” Polizzi says, adding that AlphaFold was trained on open-source protein structure databases, so keeping aspects of it private felt contrary to the ethos of the larger structural biology community. “We just hope this isn’t a slippery slope that leads to more published AI models being withheld from the public domain, where they can be vetted and used.”
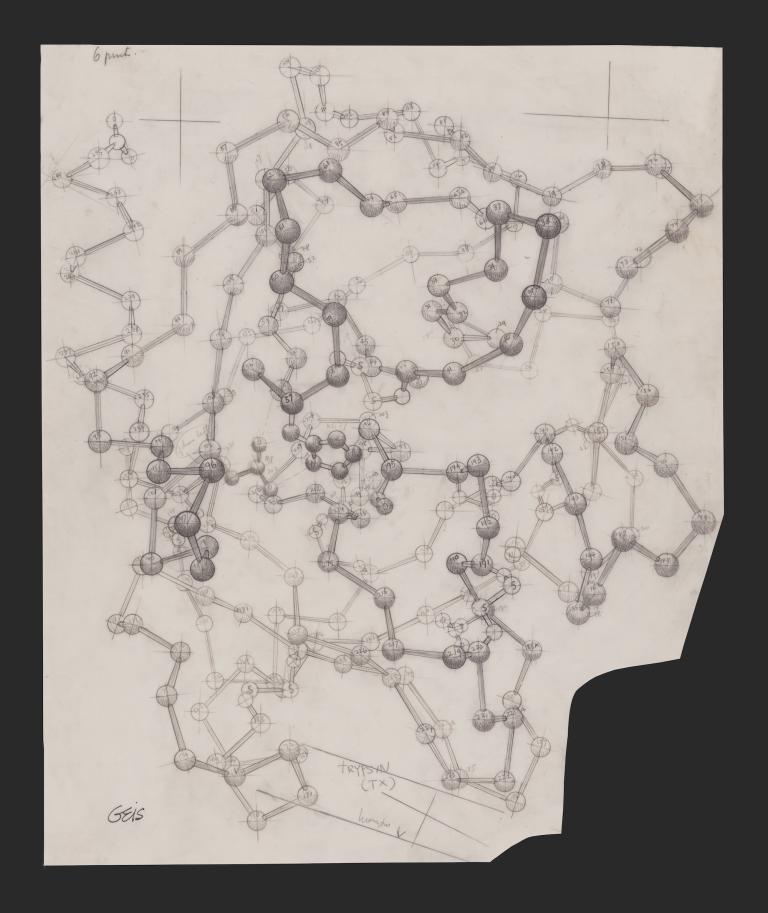
DeepMind eventually conceded, announcing that it will release AlphaFold3’s code at some point this year. But by then, Polizzi hopes, AlQuraishi, Bouatta, and colleagues will have come out with a similar model that replicates the results anyway.
Indeed, the next version of OpenFold is now in the works. “We now know that being able to use this kind of tool is not something you can take for granted,” AlQuraishi says. “The open-source component is critical and is only going to become more so moving forward.”
Problem solved?
So, in retrospect, does AlQuraishi think the initial fanfare over these types of models was warranted?
“The problem with AI and science is there is a tremendous amount of hype,” he says. “I certainly do think this is the best example of what AI can do in science. But it’s not like biology is over. There are many things it did not solve. It’s a sliver of a much bigger problem we’ve only begun to tackle.”
Plus, it’s not even safe to say the protein-folding problem is solved. One important box is ticked off: Researchers can now predict protein structures from amino acid sequences. But two other key parts of the problem — understanding the underlying physics and how proteins fold so quickly — remain unsolved.
Are we even legitimately doing science anymore, or is it something different?
“Structure is not the final story; we want to understand much more,” says Bouatta. He points out that a few years post-AlphaFold2, there hasn’t been as much progress in treating diseases as some might have anticipated. For drug discovery, he adds, a deeper understanding of protein dynamics is essential. That includes studying how proteins behave in their natural cellular environments and why certain proteins fold incorrectly — a factor linked to many neurodegenerative diseases. “This is why we should still care about the physics,” Bouatta says. “It will radically improve our ability to think about diseases and strategies for dealing with them.”
Another alluring question is whether AI models like AlphaFold and OpenFold have actually figured out something about the physics of protein folding that humans haven’t. Similar questions are being asked in other fields. Linguists are exploring how the inner workings of large language models like ChatGPT can reveal new insights about how humans acquire and process language. And computer vision AI models trained to recognize faces or objects have helped vision researchers understand how our own brains categorize what we see. “I’m optimistic about where that could go,” says AlQuraishi, “but it’s a tall order. And it will certainly require open-source models.”
Bouatta and AlQuraishi have already started peeking into the inner workings of OpenFold, examining the shapes of the structures it builds during its training to figure out how it learns. What they’ve found is a bit bizarre. For instance, instead of following steps of folding as they happen in nature, the model starts by creating one-dimensional views of folded proteins, followed by two- and three-dimensional views. The model doesn’t necessarily need to know the basic physics to do its job of piecing together the protein structures. But Bouatta argues that the more that physical knowledge is infused back into these deep learning systems, the better they’ll work — and “the better we’ll learn how they are learning.”
AlQuraishi agrees that humans do know fundamental facts about the physics of folding that the models miss. But he also has been surprised, over and over again, by how successful deep learning can be at making predictions with very limited prior knowledge of things humans have worked so tirelessly to understand.
In fact, it’s been slightly disheartening. It gives him a nagging feeling about AI that he just can’t shake. If we can predict how proteins fold without understanding how they do it, “are we even legitimately doing science anymore, or is it something different?” AlQuraishi muses. “In the past, what drove us was understanding. With this wave of AI, we’re maybe losing some of that. We’re able to get the practical benefits, but we’re not necessarily gaining intellectual benefits.”
He stays optimistic by considering the practical benefits he expects in his lifetime. Like gaining a full picture of the molecules inside cells at the atomic level. Knowing not just how single proteins behave but also how constellations of them join forces to function. Creating drugs that are like tiny machines that travel into cells to make tweaks. Building new proteins and new cells with specific functions. “These will become possible because of the advances we’re making today,” he says.
Previous generations of scientists have also seen similar seismic shifts. The quantum revolution in the early 1900s gave scientists a predictive tool that was remarkably accurate. It paved the way for nuclear energy, computing, and nuclear weapons. “But it left us with some questions about reality that … we haven’t really resolved,” AlQuraishi says.
“I think we’re seeing a transformation of science that is probably going to end up being as profound as the quantum revolution,” he says, “not just in terms of the scientific models and theories, but how we do science itself.”
Molly McDonough is the associate editor of Harvard Medicine magazine.
Images: Geis illustrations used with permission from the Howard Hughes Medical Institute (www.hhmi.org). All rights reserved. Myoglobin model image courtesy of Science Museum Group Collection, © The Board of Trustees of the Science Museum. AlphaFold2 image courtesy of Google DeepMind and EMBL’s European Bioinformatics Institute. Photo of Nazim Bouatta by John Soares. Photo of Mohammed AlQuraishi by Nicole Pereira.