Can AI Make Medicine More Human?
The history of tools used to support clinical decision-making offers clues to the future of medicine in the age of generative AI
- 15 min read
- Perspective
In The Doctor, an 1891 painting by Luke Fildes, a physician attends to a sick child.

In The Doctor, an 1891 painting by Luke Fildes, a physician attends to a sick child.
Growing up as a nerdy child in the 1980s and ’90s, no television show shaped my worldview more than Star Trek: The Next Generation. While exploring strange new worlds, the bridge crew of the starship Enterprise frequently came into conflict, which they generally resolved with diplomacy, reason, and a fundamental acceptance of human (or alien) dignity. The role of technology, especially the computer, was to enhance — but not replace — this humanity. This was equally true for Dr. Crusher, the medical officer on the Enterprise. For her, the computer was a powerful diagnostic assistant, always available at her command — similar to how I might use a stethoscope in my job as a doctor today. And as with my stethoscope, there was no tension between doctor and computer — it fundamentally augmented her abilities.
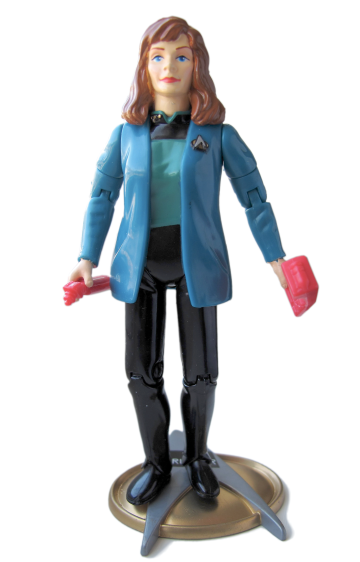
It is an understatement to say that this is not the experience of most doctors today. Rather, the computer has become a scapegoat for all that ails the practice of medicine. From the patient perspective, visits have been reduced to sitting in an exam room as the doctor stares at a computer screen, occasionally glancing over the top of the monitor while tapping on the keyboard. And for doctors, multiple studies have shown that we spend most of our day either writing or reading notes on the computer rather than interacting with the patients described in those notes, time that increasingly bleeds into homelife — something that we in medicine euphemistically call “pajama time.”
Since the fall of 2022, when the technology company OpenAI released ChatGPT to the world, the discussions of how artificial intelligence will reshape medicine have sometimes sounded like something out of Star Trek. In fact, such claims are far from new. Attempts to use technology to enhance doctors’ clinical reasoning skills — or sometimes to replace doctors altogether — are as old as modern medicine itself. As a physician and a historian of medical epistemology, I have both studied and experienced firsthand the promises and shortcomings of these technologies.
If you had asked me two years ago whether it was likely that AI would play a significant role in clinical reasoning any time in the near future, I would have said no. In fact, just a few months before the release of ChatGPT, I published my first book, an intellectual overview of medical history, and in the final chapter I discussed machine learning and new AI technologies. Although I was optimistic about the potential of these technologies, I felt that proponents of AI drastically underestimated the complexity of diagnosis and the challenges that AI systems faced.
But I was intrigued enough that over the past two years I have added AI researcher to my list of professional identities. What I’ve found has changed my outlook on the promise of AI to improve clinical reasoning and, more broadly, on the potential role of technology in medicine. Even as I remain anxious about how AI will be implemented in clinical settings, I am also surprisingly hopeful about a future in which it will augment the abilities of physicians and allow us to focus on the uniquely human aspects of medicine.
From leeches to punch cards
My optimism stems in part from my study of past attempts to use technology to enhance the reasoning of physicians. These attempts can be traced back to post-Revolutionary France, where the physician Pierre-Charles-Alexandre Louis pioneered the idea of analyzing structured data to glean insights beyond the reach of any individual clinician, starting with the study of leeches.
Bloodletting, especially via leeches, was in vogue in 1820s Paris. Influenced by superstar physician François-Joseph-Victor Broussais, known as “le vampire de la médecine,” patients would have dozens of the worms placed on their bodies, draining up to 80 percent of their blood volume. Soldiers receiving the treatment were said to appear to be wearing chain mail from all the shimmering leeches covering their bodies.
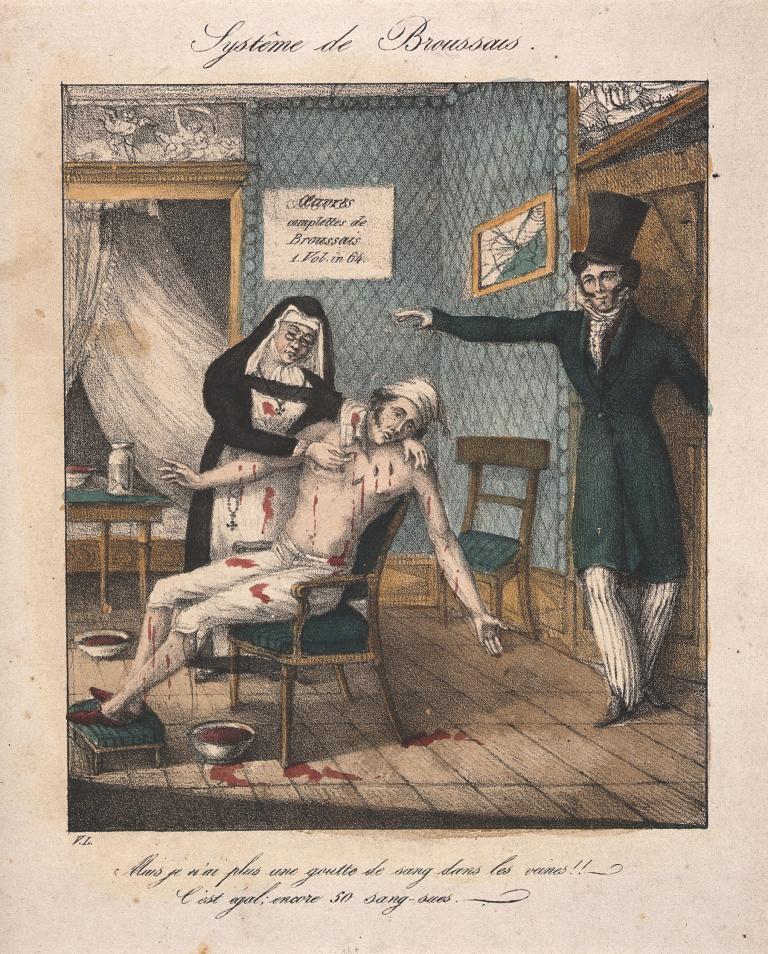
No one really doubted that leeching worked, but for unknown reasons, Louis — now generally considered the first proto-epidemiologist — decided to test the efficacy of leeching by analyzing discrete data points from multiple patients to look for trends, which he called the “numerical method.” He compared records of patients with pneumonia who were bled early in their treatment to those who were bled later and found that the patients who received “life-saving” bloodletting early in their course died at a far higher rate. Although Louis’s findings did not make an immediate impact, he was the first to realize that large amounts of organized information could, if analyzed properly, provide insights that no human ever could.
Over the following decades, Louis’s ideas became mainstream, with routine collection of vital signs and causes of death, as well as a proliferation of diagnostic tests. By the early twentieth century, reformers dreamed of using machines to automate the collection of all this data. The earliest reference I’ve found to the concept of what we would now call a medical artificial intelligence comes from the writer George Bernard Shaw, who in 1918 imagined a diagnostic machine similar to the counting machines that had changed finance.
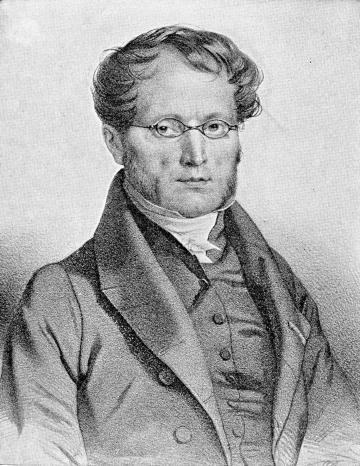
“In the clinics and hospitals of the near future,” Shaw wrote, “we may quite reasonably expect that the doctors will delegate all the preliminary work of diagnosis to machine operators as they now leave the taking of a temperature to a nurse, and with much more confidence in the accuracy of the report than they could place in the guesses of a member of the Royal College of Physicians.… From such sources of error machinery is free.”
The routine collection of data to take better care of patients permeated the medical zeitgeist, including in medical education. At HMS in the early twentieth century, Walter Cannon and Richard Cabot introduced a new didactic structure, called the clinicopathologic conference — commonly referred to as a CPC. Adapted from legal education, this new method of teaching presented learners with a “mystery case” with all the information already collected and collated. Each case followed the reasoning of experts as they developed a final diagnosis. The implication was clear — medicine was fundamentally a data-sorting task. If enough information was available and organized properly, a diagnosis would inevitably follow. CPCs soon spread around the world, and they are still published in the New England Journal of Medicine to this day.
The technological and mathematical advances fueled by World War II moved solutions of the sort Shaw imagined from fiction to reality. Inspired by attempts of the U.S. Army to screen draftees during the war effort, Cornell psychiatrist Keeve Brodman developed a systematized list of symptoms, now called the review of systems, which most people have dutifully filled out for their doctor or dentist. Brodman was also the first to describe the concept of an algorithmic doctor, in 1946, the year electronic computers were invented.
In the 1950s, after systematically going through hundreds of CPCs published in the New England Journal of Medicine, Robert Ledley and Lee Lusted — arguably the founders of medical AI — described a process by which the new fields of epidemiology and biostatistics would soon replace traditional clinical reasoning. Patients would give their history on punch cards, and a computer system would print out a list of diagnoses in order of probability. As information from additional tests was included, the probabilities would change, until eventually the computer reached a final diagnosis.
There was an incredible sense of optimism that diagnosis would soon be done entirely by computers, and, indeed, a steady drip of innovations seemed to support this outlook. There were drug-interaction checkers, expert systems that could pick antibiotics to treat almost any infection, and decision-support tools that could diagnose appendicitis on submarines. Then there’s my personal favorite: INTERNIST-I, an AI system designed to solve CPCs better than any individual human could by mimicking the diagnostic powers of a single person, Jack Myers, the chair of medicine at the University of Pittsburgh at the time.
To some extent, many of these systems actually worked in the real world, such as AAPHelp, a computer system that assisted in the diagnosis of appendicitis. In a multicenter randomized controlled trial of AAPHelp, negative laparotomies fell by almost half, error rates decreased from 0.9 percent to 0.2 percent, and mortality fell by 22 percent. But even when they worked, these systems were only effective in limited domains, such as for patients with acute abdominal pain in the case of AAPHelp. The larger dream of a generalist AI doctor fell by the wayside, replaced by the more limited goal of providing clinical decision support, and the field of AI in general fell into what is commonly referred to as an AI winter. In fact, a review of diagnostic AI systems from 2012 shows minimal gain in performance over the systems of the late 1980s.
Understanding the clinical mind
Even as progress stalled on medical AI, the field of clinical reasoning saw an important shift with the adoption of a new framing — cognitive psychology, which, like computer science, had emerged from the cybernetics movement of the 1950s. Inspired by the work of psychologists Daniel Kahneman and Amos Tversky, a new generation of researchers sought to gain a better understanding of the human mind, in some instances in order to build AI systems and in others to improve the training of doctors. They learned that medical diagnosis is subject to many of the same psychological principles as other domains of human decision-making. Much of what appeared to be interrogation in the style of Sherlock Holmes was actually what we would today call System 1 thinking — fast, efficient heuristics, constrained by the same biases and failures of other System 1 processes. For example, studies in the emergency department have shown that doctors are less likely to test for a blood clot in the lungs if a history of heart failure is mentioned, a phenomenon called anchoring bias, in which early information is valued more than later pieces.
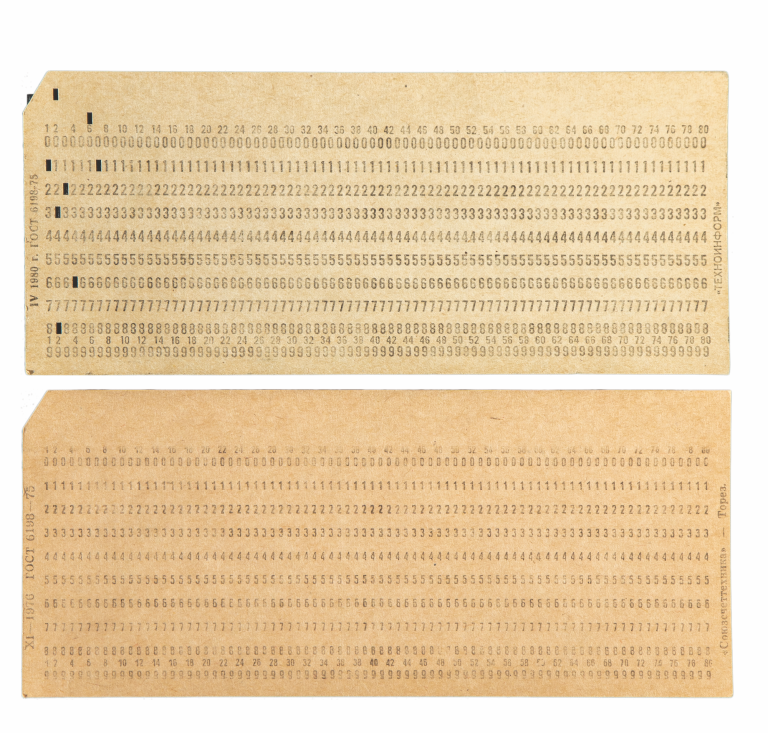
Humans, it seemed, did not think like computers, and doctors were no different. The calculation of probabilities was only a small part of how doctors formed a diagnosis. It was no wonder that the contemporaries of Pierre-Charles-Alexandre Louis couldn’t make the statistical leap to understand that bloodletting was killing their patients. Their brains simply didn’t function that way. These insights helped explain, at least in part, the failures to create an AI that could perform clinical reasoning — the early systems were based on a faulty understanding of how doctors reason. If AI systems were to be trained to think like doctors, mathematical probabilities were clearly not the way forward.
When I came of age as a physician in the early 2010s, this cognitivist understanding of clinical reasoning was dominant. One of the most important precepts of our field was that clinical decision-making was driven by the quirks of how human brains store and access information. It was almost in the realm of science fiction to think that a computer could model that messy reality. So, if you wanted to improve clinical reasoning and diagnosis — and who wouldn’t, given the incredible burden that missed or delayed diagnosis has on our patients — the focus was on educating human doctors.
But at the margins of the field, AI continued to be part of the discussion. Advances in computer power, among other factors, helped researchers exit the AI winter, and new types of machine-learning algorithms were increasingly used in medicine. These were algorithms with very specific uses, such as predicting whether a patient in the emergency department might have sepsis. And while they had significant limitations — and often dramatically underperformed when implemented — their potential spurred the U.S. Food and Drug Administration to create a regulatory pathway for such technologies. By the late 2010s, we in the diagnosis world again spoke of AI as a technology that might be able to help physicians and patients, though the timing was always projected to be at some point comfortably in the future.
From language, reasoning?
While I was likely more aware of these technologies than most physicians in the field of clinical reasoning, I’m rather embarrassed to say that large language models (LLMs), machine-learning algorithms that take human text inputs to create realistic-sounding text outputs, were not on my radar at all for having an impact on clinical reasoning. I had used GPT-3, the model preceding ChatGPT, almost a year before ChatGPT was released publicly, but while it had an eerie ability to produce poignant and sometimes absurdist poetry, there did not seem to be anything inherent in language and the association between words that would allow such a system to assist in diagnosis.
When OpenAI released ChatGPT to the public in the fall of 2022, I tested it with clinical cases to assess the model’s clinical reasoning. And while it was creative and showed what seemed to be flashes of insight, it frequently made up information (referred to as hallucinations) and its context window (the amount of text it could process in any single prompt) was too small to be useful to a doctor.
Then, in March 2023, OpenAI released an updated version of ChatGPT based on a more powerful model, GPT-4. I immediately stress-tested it with a complex clinical case — and was taken aback. There were no obvious hallucinations, and the model’s reasoning and diagnoses seemed to mimic those of an expert physician.
With just basic prompting and my exact thoughts from when I was managing the patient, the model provided a comprehensive differential diagnosis.
Next, I took a case of my own where I initially made an incorrect diagnosis, which was later corrected. I took my summary of the case, removed all identifying information, and gave it to ChatGPT, instructing it to provide a list of potential diagnoses. As I read the model’s response, I realized I was looking at something new in the history of diagnostic AI. With just basic prompting and my exact thoughts from when I was managing the patient, the model provided a comprehensive differential diagnosis. The first diagnosis the model suggested was the correct, actual diagnosis that I had been wrong about. The second was what I had thought the patient had. What if I had had this tool six months earlier when I missed the diagnosis? How would it have changed the care of my patient?
Though I was initially impressed by ChatGPT’s apparent clinical reasoning abilities, I wasn’t going to be swayed by a single anecdote. I adapted Ledley and Lusted’s chosen methodology of using CPCs and ran an experiment, eventually publishing the results in JAMA. The study found that GPT-4, with no additional training on medical resources, had an emergent ability to make helpful differential diagnoses equivalent to or better than any system that had been developed previously — and far better than even the best human physicians.
A blizzard of similar studies confirmed these results. The more advanced LLMs had what appeared to be an understanding of the connections between diseases and how their likelihood varied depending on test results and other factors, a level of understanding superior to that of humans, despite not having access to the real world or localized epidemiologic information. The models performed well not just on CPCs but also on real cases taken directly from medical records. Patients started using these tools as well, in some cases to correct missed diagnoses. The models could present reasoning better than humans, make more accurate diagnoses, and even, in an impressive study by researchers at Google, collect a clinical history directly from a patient in a true blinded Turing test.
How is this possible? How can an AI algorithm that basically uses a huge corpus of text to predict the next word in a sentence possibly outpace humans in diagnostic ability? The answer likely lies in the fact that the way these models make text predictions is remarkably similar to our understanding of how physicians make decisions, an understanding that grew out of the cognitive psychology movement.
Doctors store diagnostic information in semantic groupings that we call scripts. When we see a patient with acute shortness of breath, it activates diagnoses such as a pulmonary embolism or a massive heart attack. A patient with chronic progressive shortness of breath brings up a completely different list of diagnoses, such as heart failure or interstitial lung disease. As doctors gain experience, we refine these scripts. This is remarkably similar to the statistical associations between the strings of tokens that undergird LLMs. Although LLMs lack real-world experience, they also encode far more knowledge than I will ever have. And perhaps most importantly, they never have to admit five patients in a row at 2 a.m. while fueled only by a pot of coffee.

This gets us to another reason these technologies have so much promise. As Louis’s study of leeches showed, and as an abundance of research has confirmed, human clinicians — myself included — are deeply flawed. The cognitive load of modern clinical environments is far too much for any human being to realistically manage. The full text of the charts of all my patients when I come on service with my residents is longer than Melville’s Moby Dick. Doctors are interrupted every few minutes by pages or secure chat messages. We are subject to the same cognitive biases that affect all humans, such as anchoring bias and confirmation bias, where we give greater weight to evidence that supports what we already think. To adapt George Bernard Shaw’s phrase, from such errors, LLMs are free.
Or are they? There are doctors and researchers I greatly respect who think I’m deluding myself. Aren’t these models just “stochastic parrots” cosplaying a doctor for my benefit? And what about the persistent concerns about racial, gender, and ethnic biases encoded in their pretraining data, concerns that have been validated by multiple rigorous studies?
Then there’s perhaps the most serious concern: hallucinations, incorrect statements that are an artifact of the way models make their text predictions. There are methods to mitigate hallucinations, and we’ve already seen improvements, but there is no reason to think this problem will ever be completely solved.
A strange new world
Despite these real and serious drawbacks, my research on AI models, as well as my work as a physician and historian, leave me optimistic about what is to come. I can’t help but hope that AI moves us at least somewhat closer to the Star Trek dreams of my childhood — a system that can make humans more human and help doctors take better care of our patients.
In the short term, this would most likely consist of a second opinion consult service. Just as data suggest that human second opinions improve clinical care, an AI second opinion placed as an order in the electronic health record could do the same. This work is still in its infancy, and anyone who says differently has something to sell. Important questions remain. At which point in the diagnostic process is an opinion most helpful? How can outputs be monitored to protect patient safety? Which patients would benefit the most? But building such a system right now requires only careful study — we already have the technology.
Never before have I been so hopeful about a future where technology truly helps me be a better human being.
Looking ahead a few years, I can see the possibility of having hundreds or even thousands of AI agents deployed across the health care infrastructure, reading our notes, giving us feedback, recommending personalized education, and even listening in on our encounters with patients, prodding us to ask better questions or consider a diagnosis we might have forgotten.
It sounds like science fiction, but this future already exists. I’ve used it. I recently took care of a pregnant patient with abdominal pain so severe that she landed in the hospital. The specialists were stumped, especially because her pregnancy meant that many of our advanced tests could not be used. Her lab values were worsening, despite efforts to dig deep into the medical literature and consultations with multiple specialists. My patient was despondent. Finally, while pacing around her room late one evening, I asked her if she minded if I consulted an AI. She enthusiastically agreed, and I opened the ChatGPT app. Being careful not to disclose any protected health information, she and I had a conversation with the model, going over our questions. The AI gave a thorough list of everything that I could be missing, and then the patient and I went over each possibility to talk about what fit her case and what did not.
In the end, my initial hypothesis was right. My patient improved and was discharged home. ChatGPT, of course, was also right. But what stood out to me about the encounter was how using the technology affected how my patient and I felt about her care. It increased our confidence and engaged us more fully as people. In other words, it made our encounter more human.
Large language models are not a panacea for medicine. In fact, I’m pessimistic about how some of these technologies are currently being rolled out. And these models will not fix larger problems, such as the cost of care or access for vulnerable populations.
But never before have I been so hopeful about a future where technology truly helps me be a better human being instead of trying to convert me into a data entry clerk whose primary job is to collect information. That’s a strange new world that I can’t wait to explore.
Adam Rodman is a general internal medicine physician, educator, researcher, and writer at Beth Israel Deaconess Medical Center and an assistant professor at HMS. He lives in Roxbury, Massachusetts, with his wife and two sons.
Images: The Tate Museum (The Doctor); creativecommons.org (Dr. Crusher); Wellcome Collection (bloodletting and Louis); Getty Images (punch cards); John Soares (Rodman and residents)