The Importance of Nuance
Artificial intelligence may seem objective, but it's subject to human biases
- 15 minute read
- Feature
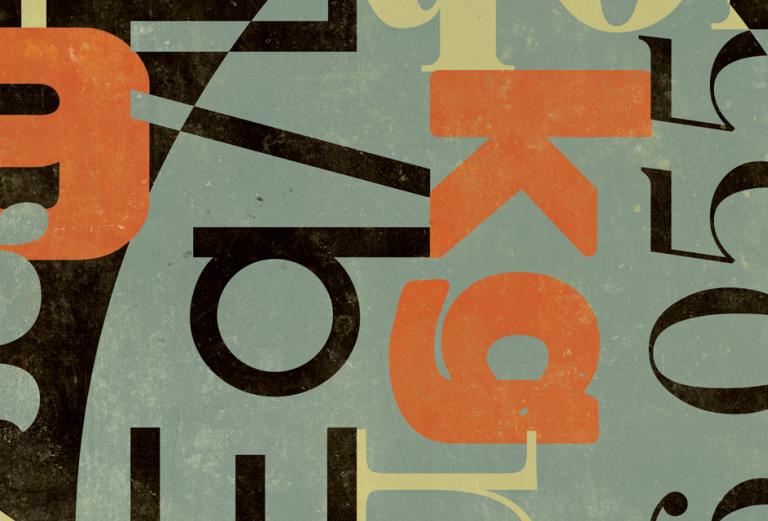
In 2016, researchers in Heidelberg, Germany, built a sophisticated computer model, called a neural network, to identify melanomas based on clinical images. They fed it more than 100,000 photographs of lesions labeled “malignant” or “benign” and let it reverse-engineer its own methods for differentiating them.
The team then invited dermatologists from around the world to compare their diagnostic expertise to the model’s. Provided with a new set of images and clinical data, the fifty-eight dermatologists, including thirty experts, from seventeen countries accurately diagnosed 88.9 percent of the melanomas and 75.7 percent of benign moles. The neural network detected 95 percent of the melanomas and 82.5 percent of the moles.
When the study was published in Annals of Oncology, it was hailed as another example of the promise of artificial intelligence in medicine. What remained other than to train the model on more complex cases and debate whether and how to one day incorporate such a tool into clinical practice?
Yet, down in the limitations paragraph of the paper, a problem became apparent: More than 95 percent of the images used to train the model depicted white skin.
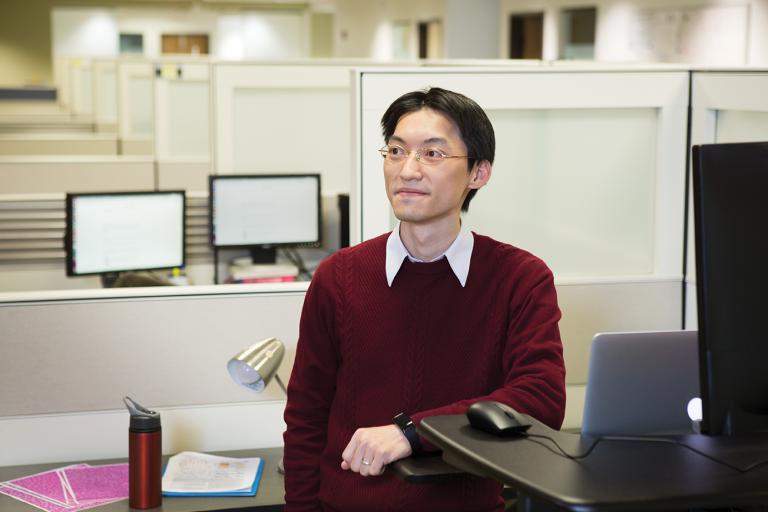
If the model were implemented in a broader context, would it miss skin cancers in patients of color? Would it mistake darker skin tones for lesions and overdiagnose cancers instead? Or would it perform well?
To ensure that AI applications provide the greatest benefit while doing the least harm, it’s essential to acknowledge that algorithms, like the people who construct them, use them, and gather the data they analyze, can be biased—and that steps must be taken to identify and correct such biases.
An all-purpose tool
With its increasing power to analyze enormous data sets and make accurate predictions, artificial intelligence is poised to sweep through medicine. It has made rapid progress in certain clinical tasks, especially in analyzing images. Recent studies show that algorithms can rival or even outperform experienced clinicians in detecting abnormalities ranging from diabetic retinopathy to pulmonary tuberculosis. Models also can predict with startling accuracy outcomes such as an admitted patient’s length of stay, likelihood of in-hospital death or unplanned readmission, and diagnosis at discharge.
Meanwhile, algorithms designed for basic science are making headway in difficult endeavors such as extrapolating protein structure and function from gene sequences and predicting the health effects of gene variants. In the translational realm, computational tools are learning to prioritize potential drug targets, predict drug activity and toxicity, discover new drugs and disease biomarkers, and unearth additional uses for existing drugs.
These models and others have the potential to extract new insights from data sources too vast for human minds to decipher; generate more consistent diagnoses and prognoses and deliver them faster; and save money, resources, and time that health care practitioners, for example, can spend more meaningfully with patients. For now, however, most models remain in various stages of development with a focus on improving their safety and accuracy.
Tread cautiously
“AI is smart in many ways, but it is subject to the principle of ‘garbage in, garbage out,’ ” says Kun-Hsing Yu, an instructor in biomedical informatics in the Blavatnik Institute at HMS. “If the input has a systemic bias, the model will learn from that as well as from actual signals.”
Biases can enter at any stage of AI development and take many forms, from analysis of skewed or insufficient data to the confirmation bias that leads a radiologist to agree with an algorithm’s false-negative findings and thus miss a lesion in a patient’s x-ray. Demographic biases alone span gender, race and ethnicity, age, income, geographic location, primary language, lifestyle, and BMI; failure to detect any of these when building or implementing a model can replicate or exacerbate disparities in patient care.
“If you’re training any algorithm to make decisions, you’re incorporating the structure of the way things work today or how they worked in the past,” says Brett Beaulieu-Jones, an HMS research fellow in biomedical informatics. “If you’re not controlling for and getting ahead of biases, you’ll perpetuate them.”
"Evaluate how representative your data set is. Perturb the data, see if your model is sensitive to demographics."
Biases may often be unintentional, but they could also be introduced deliberately. Some researchers point out that AI applications in hospitals could be designed to prioritize so-called quality metrics or make recommendations that financially benefit the AI manufacturer, a drug company, or the institution without clinicians’ or patients’ knowledge.
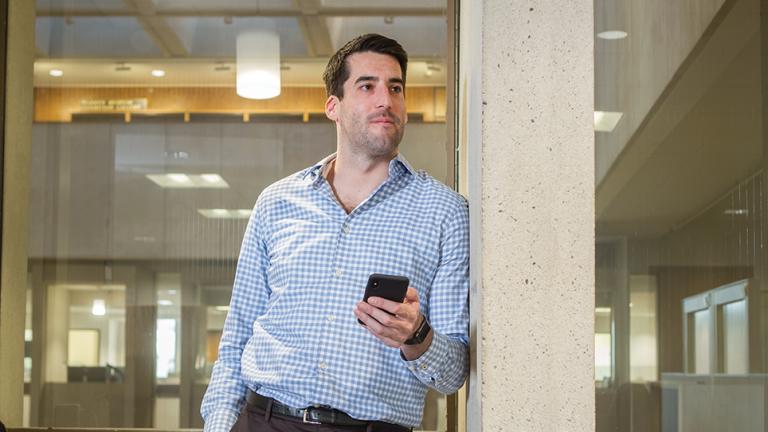
Overlooking bias in medical AI invites serious consequences. Recommendations based on biased models or inadvertent misapplications of a model could result in increases in illness, injury, and death in certain patient populations. Biased models could waste time and money in the lab and lead researchers on wild-goose chases when they’re trying to translate fundamental discoveries into new treatments. And they could erode what fragile trust the profession or the public may place in medical AI.
Just ask
AI researchers really buckled down to address the problem of bias within the past few years, say Yu and Beaulieu-Jones. Groups such as the AI Now Institute at New York University, a research center investigating the social implications of artificial intelligence, have sprung up to hold the field accountable for addressing issues such as bias and inclusion.
To date, the United States has no requirements to test for bias in AI and no standard for determining what bias is or whether it exists. Thus, researchers across disciplines are on their own when establishing best practices and raising awareness of pitfalls.
To spot bias, they agree, people first need to be aware that it may exist. That requires correcting misconceptions that machines are objective and infallible as well as admitting to the possibility of individual, institutional, professional, or societal biases.
The first place to look for bias is in the data sets used to “teach” AI models before those models can apply their lessons to new cases. Since AI has a bottomless appetite for data, researchers are realizing they must ensure that the data are of the highest quality and fully represent the patients, or the proteins, the resulting model will be applied to.
“If you think your data set is perfect and you don’t ask what can be wrong, what are the biases, you create more bias,” says behavioral scientist and lawyer Paola Cecchi-Dimeglio, a senior research fellow at Harvard Law School and the Harvard Kennedy School. “But if you pause, you can prevent the reinforcing of bias.”
AI developers and those who use the tools they build can ask where the data originated and what biases it might contain. Was information pulled from a public repository of genome sequences? If so, chances are that more than 80 percent of that DNA came from people of European ancestry, increasing the likelihood that a model trying to uncover disease-associated gene variants would reach faulty conclusions when applied to other populations.
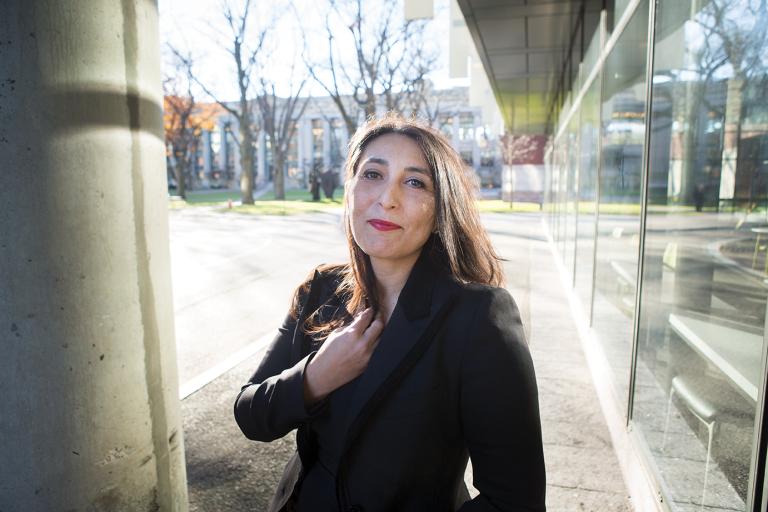
“Evaluate how representative your data set is,” says Yu. “Perturb the data, see if your model is sensitive to demographics.”
If a weakness is detected, researchers can gather more-robust data sets. Crowdsourcing, for example, can provide new data that includes groups underrepresented in the original samples. The Heidelberg research team ended up opening its skin-cancer image banks to contributions from around the world.
Whereas now “there’s almost an incentive to have a homogeneous data set to achieve greater statistical power,” funders and policymakers could help prevent bias by supporting researchers in collecting more diverse samples, says Beaulieu-Jones.
An algorithm of one’s own
Those who can’t feasibly generate new data still have options. Some researchers can pull from multiple data sources to try to reveal or balance each one’s biases, says Beaulieu-Jones. Some may be able to start training their model on a large, less representative data set, then fine-tune it on a smaller, more specialized one. Others might perform statistical operations that can improve imperfect data, such as weighting samples differently or using estimated values to compensate for missing data, a method called imputation.
What data scientists call “missingness” poses one of many challenges for those seeking to tap the firehose of information collected in electronic health records. In one study, Beaulieu-Jones and colleagues found that the EHRs for most participants in a group of clinical trials for amyotrophic lateral sclerosis (ALS) were missing 50 percent of relevant data points. Not only do patchy data hamper analysis, data that are systematically missing can drive bias. Consider the problematic outcome if, for example, an algorithm is programmed to maximize accuracy by ignoring incomplete records: the patients with fewer tests or whose medical histories are scattered across multiple EHRs often are those with mental health conditions or lower incomes.
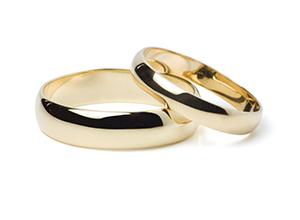
That’s where imputation can help. It’s a specialty of Beaulieu-Jones’s, who, with HMS colleagues, considers EHRs a more demographically comprehensive source of data about neurodegenerative diseases than clinical trials, which trend white, male, young, and affluent. In the ALS paper, he found that conducting imputation to bolster EHR data and reduce bias didn’t harm the algorithms’ ability to predict patients’ disease progression.
In a separate study in JMIR Medical Informatics, he and colleagues compared twelve imputation methods using the EHRs of 602,000 patients from Geisinger Health System in Pennsylvania. The team was then able to advise researchers with less technical expertise on how to assess missingness in their own data and when and how to conduct repairs, such as imputation, on EHRs. The team’s code and methods are publicly available.
There’s another opportunity to catch biases after an algorithm has processed the data and delivered its conclusions. Machine-learning algorithms—those that develop their own prediction rubrics from the information they’re fed—identify associations, only some of which are causative. As with any research endeavor, Yu and others stress, it’s critical to think about confounding factors when building and using AI models. In EHRs, potential confounders abound in the guise of not only missing data but also patient zip codes, differences in which patients get lab tests, diagnostic codes that are chosen for insurance reimbursement, and date of visit standing in for date of onset of condition.
And that’s not even getting into the fact that race, gender, and biology aren’t as clear-cut as traditionally defined.
“We need to do more careful analyses and more causal analyses,” says Yu. “AI is so complex that we may need a more sophisticated understanding of, and language for, cause and effect.”
If all other attempts to control for bias fail, say Yu and Beaulieu-Jones, researchers and AI developers can at least state the study’s limitations so others can act accordingly.
Describing the elephant
It’s even harder to assess bias when details are lacking about data provenance or when algorithms are either too complex to understand or concealed by the designer for proprietary reasons.
“Black box” algorithms that prevent insight into how tools make decisions are a major concern in the AI field today. Some turn to legislation and advocacy. Cecchi-Dimeglio joins many others in wanting more regulation in this country around ethics and transparency. Samuel Volchenboum, an associate professor of pediatrics and director of the Center for Research
Informatics at the University of Chicago, argued in a coauthored Harvard Business Review article that government institutions such as the U.S. Department of Health and Human Services need to prevent medical data sets from being privatized, as credit scores were.
Others, including Beaulieu-Jones and Yu, are trying to pry open black boxes to uncover hidden biases or other issues that could affect patient safety or research quality. In cases where they can’t peek inside, they look for other ways to gauge how the algorithms might be operating.
“Opening up the black box is hard,” says Yu. “Without opening it, we try to at least grasp the basic concepts, such as what the algorithm is paying attention to.”
Yu is part of an international research consortium training machine-learning algorithms to interpret histology slides. The group’s goal is to investigate the dogma that pathologies present in consistent ways at the cellular level across humanity. For an AI application that interprets visual data, as this one does, he might apply what’s known as an attention map to discover whether the algorithm spends more time analyzing the area of interest, such as a tumor—or something else.
“An algorithm might accurately distinguish cats from birds, but it might be doing so by identifying trees in the background, because birds are more often found outside,” says Yu. “You want to know how it’s arriving at its conclusions.”
Similar methods prove useful even when the inner workings of AI applications aren’t secret. Many neural networks consist of more than one hundred so-called layers that together process tens of millions to hundreds of millions of parameters, says Yu. He might look at the inputs and outputs between the first few layers to see how they detect lines, edges, circles, and dots in an image, but the higher-level features remain out of reach.
On the opposite end of the spectrum from black-box engineering, many AI developers practice open-source coding. Transparency, however, doesn’t mean bias is automatically identified or removed, cautions Beaulieu-Jones.
Multiple choice or essay
Accessible or opaque, simple or convoluted, the key to reducing AI bias is to test, test, test. Algorithms must be built and tested in diverse environments, say UCSF researchers in an article in JAMA Internal Medicine in November. Chief among the AI Now Institute’s exhortations is to treat new AI applications like drugs entering the market, ensuring that they undergo rigorous scientific testing followed by continuous monitoring of their effects.
The good news is people don’t need a degree in computer science to use AI responsibly. A basic education in how algorithms work can help users understand where bias might creep in, how to ask an AI application the right questions, how to apply the results in appropriate ways, and when to use or forego a particular piece of software in their work. Along with Yu’s and Beaulieu-Jones’s work to demystify aspects of AI, high-level organizations, including DARPA, are funding projects to raise user literacy.
The lift from a rising tide
Data sources and testing environments aren’t all that can be diversified in the pursuit of reducing bias. Creating more interdisciplinary teams that mix computer scientists, bioinformaticians, clinicians, researchers, and epidemiologists would raise the likelihood of unbiased AI results, wrote the UCSF team. Others call for broadening the demographic diversity of the teams that collect data and recruit for studies, and for funding agencies to more equitably award grants to such researchers. The teams that build AI tools could use improvement as well; the technology sector lags behind other industries in diversity, according to the U.S. Government Accountability Office.
Cecchi-Dimeglio uses AI when she consults with organizations, including those in the health care and pharmaceutical industries, to improve diversity inclusion. She has found that her scientific approach goes over well in the medical arena because it begins with data analysis and evidence-based decision making, then moves to what she calls “nudge” interventions: testing changes one at a time and measuring the results until outcomes improve.
“It’s not just a gut feeling; this is what the data tell you,” she says. “We all are biased, but you’re not telling someone that. You’re able to shift to a productive conversation.”
Cecchi-Dimeglio isn’t the only one turning to AI to reduce bias in medicine. In September, Google released an open-source, visually based algorithm called the What-If Tool that allows users to assess the fairness of machine-learning models without needing to write code; Microsoft and IBM report that they are also working on automated bias detectors. More broadly, Beaulieu-Jones points out that since algorithms learn from current practice, they can surface existing biases. “That’s one area where AI can be of help,” he says.
There are even murmurings about training algorithms on human ethics alongside technical tasks. IBM says it is working to incorporate “computational cognitive modeling, such as contractual approaches to ethics,” into algorithmic decision making.
How good is good enough?
As medical AI evolves, the community faces difficult questions. Who decides what is fair? How much bias is acceptable? Do algorithms need to be perfect, simply better than people, or merely as good?
AI models that perform as well as or better than an average practitioner could benefit regions that are short on specialists, wrote Yu and colleagues in Nature Biomedical Engineering in October. Minimizing algorithmic bias would be critical for ensuring that resource-poor communities don’t get handed off to algorithms that provide substandard care.
Experts emphasize that the end game for AI isn’t to replace clinicians, researchers, or other human specialists. Nor could they, says Yu, who rests easy knowing that physicians will always have empathy and human touch on their side.
“As an MD by training, I don’t want to be replaced by AI,” he says. “But I’m not worried. It’s more like doctors who don’t use AI will be replaced by those who do.”
Stephanie Dutchen is a science writer in the HMS Office of Communications and External Relations.
Images: Traci Daberko (top); John Soares; Cemay/Essentials/Getty (rings)